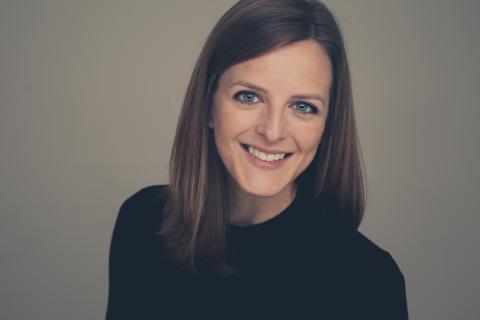
June 10, 2024
We're delighted to congratulate SCARP Assistant Professor Julia Harten for receiving research funding from the 2023 New Frontiers in Research Fund Exploration Stream, and showcase the revolutionary new research she has planned for her approved project.
About NFRF
The Government of Canada's New Frontiers in Research Fund (NFRF) supports world-leading interdisciplinary, international, high-risk / high-reward, transformative and rapid-response Canadian-led research.
This fund seeks to inspire innovative research projects that push boundaries into exciting new areas and that have the potential to deliver game-changing impacts.
About Julia Harten
Dr. Harten is an Assistant Professor and Canada Research Chair in Data Innovation for Housing and Inclusive Urbanization in the School of Community and Regional Planning at the University of British Columbia. Dr. Harten's work leverages innovative data strategies for the study of housing and sociospatial inequality, focusing on the housing strategies of marginalized people and the role of cities and housing for social mobility. Dr. Harten’s work, covering both domestic and international housing challenges, has been published in top planning journals (e.g., Journal of the American Planning Association, Journal of Planning Education and Research, Urban Studies), is regularly featured in high-impact planning and urban studies conferences, and has won multiple awards as well as funding from prestigious institutions, both in Canada (e.g., Social Sciences and Humanities Research Council SSHRC, Canada Mortgage and Housing Corporation CMHC) and abroad (e.g., Lincoln Institute for Land Policy, U.S. Dept. of Education). Notable community engagements include leading the housing needs assessment for the CMHC-funded Housing Assessment Resource Tool and consulting on rental housing need for the City of Vancouver and Immigration, Refugees and Citizenship Canada.
A little about this research
Dr. Harten (and Co-Principal Investigator Christos Thrampoulidis, Assistant Professor in UBC's Electrical and Computer Engineering) will introduce a new potential strategy for meaningful change in the housing crisis, with the potential to democratise housing development, revolutionise housing supply, and unlock a new path for social science research leveraging ethical artificial intelligence (AI).
This research focuses on the process local governments set up to decide whether a proposed housing development should be allowed to move forward. Deliberations and decisions are documented and public, but enormous and unstructured. Together with their team, Dr. Harten and Dr. Thrampoulidis will analyse public records leveraging the latest advances in AI to reverse engineer the key ingredients of successful development applications. What gets a project approved? What arguments work? How does council vote and who speaks up at public hearings?
In a little more detail:
Opening the Approvals Black Box:
Leveraging Large Language Models and City Council Public Records to Understand Housing Supply
Principal Investigator | Julia Harten, UBC SCARP |
Co-Principal Investigator | Christos Thrampoulidis, UBC Electrical and Computer Engineering |
Research Summary
Housing affordability is one of the most pressing issues of our time. Numerous efforts have been made to uncover the root causes and address the crisis, yet meaningful change remains elusive. We propose to investigate the housing development approvals process. As housing is tied to land, which in cities is scarce and contested, local governments set up approval processes to ensure that new developments meet community interests. In practice, however, these often become highly political, lengthy negotiations where it is unclear whether what gets approved truly serves the public good.
To open the development approvals black box, we propose leveraging recent breakthroughs in AI, particularly Large Language Models (LLMs), to analyze public records of city council meetings. City council meetings, where housing development decisions are made, are documented in text and video. This data is publicly available but effectively inaccessible due to its large volume and unstructured nature. We propose a computational pipeline that uses LLMs in partnership with human researchers with the goal to identify the key ingredients for successful development applications. To do this, we automate the parsing of Vancouver city council meeting minutes and machine-assisted transcription of video records and identify segments pertaining to housing development decision-making. Then, we employ LLMs for topic modeling to identify patterns, e.g., in the makeup of development proposals or arguments made at public hearings. Finally, we utilize LLMs for conversation-level classification to uncover key players via the analysis of power relationships, revealed in speakers' linguistic style.
By democratizing development approvals, this work has the potential to revolutionize housing supply. More broadly, it will help unlock the power of LLMs for social science research. Text data in social science is ubiquitous, but previously its labor-intensive analysis was limiting its use. LLMs have demonstrated impressive results in processing such data, but raise concerns with regards to cost barriers, transparency, their sensitivity to prompt engineering, and challenges in handling domain-specific terminology or noisy data. By subjecting LLMs to the rigorous test of applying them to a complex, real-world domain, we push the boundaries of their application spectrum and develop solutions that address their limitations.
Potential Impact
This research promises to make contributions in at least three critical areas. Firstly, unlocking the drivers of success and failure within the development approval process may be an effective, yet heretofore unexplored strategy to promote more and more diverse supply of housing. Housing is an urgent issue in cities around the world. As a result, there is a large and growing body of work inquiring into the root causes and potential solutions of the current challenges. Research on identifying housing supply constraints has largely focused on policies, laws, and regulations, in particular zoning, and the effect of political organizing such as Not In My Backyard (NIMBY) activism. The development approval stage has not been studied. It has, however, attracted the attention of Canadian lawmakers. Seeing the urgency of the crisis and the ineffectiveness of the current approach, the BC provincial government has started to implement municipal housing targets and set up strategies to ensure compliance. This involvement of higher levels of government, in what has traditionally been a local issue, is unprecedented and highlights the timeliness of and need for this research.
Specifically, identifying what leads to success or failure of housing developments at the critical approval stage could empower smaller and non-profit developers to submit proposals - actors who might otherwise be deterred by the potentially enormous costs and risks associated with the current, lengthy process. Municipalities will be interested in these findings as well, as understanding why and when development proposals fail may help them fulfill their mandate to address the housing crisis. Higher levels of government at the metro, provincial, and federal level, who have already demonstrated a renewed interest in housing supply, may also benefit from this work as it could inform future policy directions. This is especially true since we expect to uncover dynamics that are relevant for North American cities, beyond the Vancouver context.
At a broader level, this work stands at the forefront of unlocking the transformative potential of LLMs for urban planning, and more generally, social science research. The social sciences have so far been slow to adopt AI technologies even though text data have long played a critical role. With planning documents widely available and many planning-relevant exchanges happening online, there are now ample opportunities for urban planning to generate new, large text datasets and use them to garner fresh insights into enduring planning puzzles. Indeed, first studies have already shown the power of harvesting and mining big text data to study persistent planning challenges, from housing discrimination and affordability, to transportation accessibility, and gentrification. Even with these first computational approaches, however, time and labor costs have been limiting factors. The rise of LLMs holds the promise of mitigating these, but they come with their own set of costs. Beyond computational costs, accessibility, transparency, and bias are cause for concern. At the same time, these technologies enable new research endeavors: this work would previously have been infeasible due to the large volume of unstructured text data that needs to be processed and analyzed. To pave the way for the social sciences to leverage the powers of the AI revolution, what is needed is a careful study of the opportunities and limitations. Findings from the analysis of city council public records will effectively showcase the potential for AI-assisted social science.
Furthermore, this work will serve as a test case to investigate how LLMs perform as compared to more established methods, such as manual qualitative coding and conventional computational techniques such as bag-of-words representations and latent Dirichlet allocation (LDA). As a result of these comprehensive comparisons, we will be able to formulate guidelines and detailed risk-benefit evaluations, empowering social science researchers to make informed choices about the employment of LLM technologies.
Working through progression of language models varying in sophistication, compute costs, and interpretability, we can also identify tasks suited for cost-effective options and minimize the use of the largest models for challenging reasoning tasks, facilitating the use of LLMs in scalable and sustainable frameworks. Rather than replacing human expertise, we will experiment with computational pipelines that combine LLMs with human expert intervention. While we anticipate that LLMs will significantly expedite processing and textual tasks, such as topic modeling and speech emotion recognition, this approach emphasizes the critical and multifaceted role of human experts in (a) effectively prompting the LLM, (b) generating suitable examples for few-shot in-context learning, and (c) interpreting the results and transforming them into concrete insights. Finally, to address concerns about transparency and accountability and ensure broader accessibility, this work will prioritize the use of open-source models, which come with the additional benefits of reproducibility and adaptability to specific tasks.
Applying LLMs to the analysis of public records and testing the performance of various configurations will also generate valuable insights for the AI research community. LLMs have spearheaded a revolution in language processing and generated enthusiasm due to their exceptional ability to excel across diverse tasks without explicit fine-tuning. Still lacking is a systematic identification of limitations and failure modes, especially in new domains. As the landscape of available LLMs diversifies in terms of size, compute requirements, transparency, and integration capabilities, the need for frameworks that enable comparative measures is pressing.